How AI will take mobility to the next level: the example of the JULIA project
In the same way that satellite technologies have revolutionised geolocation, Artificial Intelligence (AI) is poised to significantly change the mobility landscape, challenging long-held assumptions about the limitations of computer systems in tackling complex problems. AI, particularly through machine learning (ML), enables machines to see, listen, interpret, predict, and comprehend, mimicking human capabilities. Advances in AI technology are proving that machines can perform these tasks effectively.
In the context of JULIA, a Horizon Europe project coordinated by Factual aimed at enhancing public transport’s reliability, safety, and sustainability using EU Space Data and Services, AI will be used to improve Demand-Responsive Transport and assess the safety of cycling lane infrastructures.
Streamlining Demand-Responsive Transport in sparsely populated areas
Demand-Responsive Transport (DRT) offers an innovative approach to traditional public transportation, particularly benefiting sparsely populated areas with lower demand, offering a more flexible and cost-effective alternative to fixed-route buses. NEMI, market leader in DRT solutions in Spain, leverages AI within a multivariable parametrised optimisation tool.
This AI-driven approach allows NEMI to simulate demand-responsive mobility services using various optimization algorithms tailored for shared mobility. This results in broader route coverage, improved accessibility for a wider audience, more accurate passenger information, reduced travel times, and streamlined digitalisation of public transport services.
In the context of JULIA, AI algorithms fed by Origin-Destination data provided by Galileo-enabled devices are being developed, which will be exploited to provide highly personalised bus travel solutions using Machine Learning algorithms to support mobility planning, select the most appropriate mobility offers and provide more accurate real-time pick-up and arrival information while optimising the overall operation of Demand Responsive Transit systems. AI will also be used to improve the vehicles map-matching process, where topology-based techniques will be combined with Machine Learning capabilities using similarity indicators to significantly reduce geotagging errors, using it for the more precise location of bus dynamic stops in DRT.
NEMI leverages Galileo services, the European Global Navigation Satellite System. Galileo’s precise and reliable positioning information is crucial for DRT. It enables accurate planning and real-time adjustments of routes, minimising friction between users requesting rides and the available buses that can serve their needs. This integration further enhances the efficiency and responsiveness of shared mobility services, ultimately benefiting a larger portion of the population.
Automatic recognition of cycling infrastructure attributes in safety assessments
Lane Patrol is a first-to-market software tool to map and assess the safety of cycling infrastructures utilizing the CycleRAP methodology. By focusing on evidence-based risk assessments, primarily extracted from cycling infrastructure images (or video footage), the CycleRAP model helps identifying high-risk areas without relying exclusively on past collision data. Up until now, the process of collecting and analysing the images for CycleRAP has been mainly manual, which involves significant hands-on work and can be time-consuming, plus prone to errors.
Building on computer vision, Lane Patrol is exploring techniques to automate parts of this process, simplifying, and accelerating the CycleRAP evaluation process. Deep learning models, such as deep neural networks, are a type of Artificial Intelligence (AI) capable of processing and interpreting complex data with high accuracy. At the image level, they are capable of performing complex tasks such as image classification (to determine lane types, the presence of tram rails, or whether there is light segregation, that is, if the image contains curbs, bollards, etc.); object detection (capable of detecting many types of road defects, persons, such as pedestrians, other light vehicles, etc.); object tracking (of other cyclists, pedestrians, cars, etc.); or semantic segmentation (for lane detection, including the presence of proper delineation, etc.).
All these methods are suitable for classifying the attributes of the CycleRAP method. Lane Patrol uses an AWS AI algorithm to blur faces and license plates before uploading the images to the server for further processing, therefore ensuring privacy protection. Integrating computer vision with learning algorithms allows Lane Patrol to improve over time. As more data is gathered and labeled, the system’s accuracy in classifying CycleRAP attributes will increase.
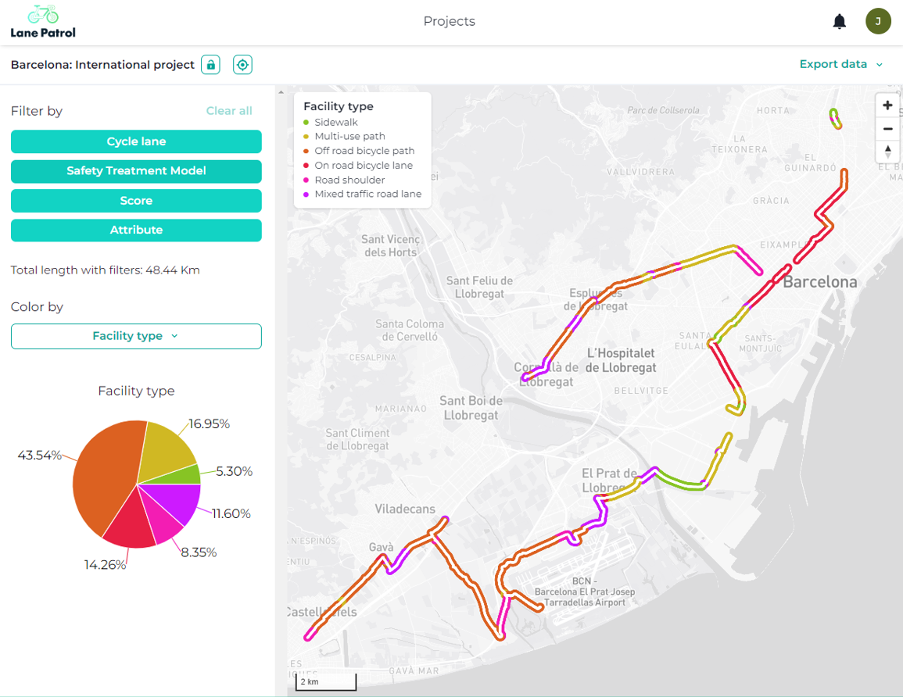
CycleRAP requires acquiring images of a cycle lane down to every 10 metres. The accurate calculation of this 10-metres distance is solely based on the positions calculated by the GNSS receiver integrated in the acquisition device (Lane Patrol smartphone app), and hence accuracy and positioning availability is key. Errors in positioning calculations can lead to distance calculation errors and consequently image results that do not meet the criteria for a successful assessment. In this scenario, the use of multi-constellation GNSS receivers with Galileo has proven to be a good approach, especially in urban environments where the number of satellites in view are constrained by surrounding buildings. This use case is also part of the JULIA project.